Whether it’s recent news or just new to you, The Data Planet serves up fascinating insights and resources ABOUT the Data analytics and BI WORLD EVERY MONTH.
Our snack-size summaries skip straight to the point.
This month’s Data Planet includes:
- The Power of Product Thinking
- Data as a Product
- AWS Data Exchange for Amazon Redshift
- Apache Spark™ 3.2 Available on Databricks
- AWS Hero Writes: "9 Things I Love About Google Cloud Identity"


The Power of Product Thinking
Product thinking is something you should know about. The story of the Juicero Press illustrates it well. Juicero was a juice-making machine where you’d put a pre-packaged fruit packet in the machine, and it would squeeze out juice. It was like a Keurig, but for juice.
By all accounts, Juicero was marvelously engineered: Wi-Fi enabled, built in QR-code scanner, machined aluminum frame, and a high-powered drivetrain. Yet, despite $120 million in investment, the company folded after two years.
Why? First, the machine was too expensive at $700 a unit. Second, people soon discovered they could just as easily squeeze the fruit packets by hand. Juicero was a product in search of a problem.
Product thinking takes the opposite approach. You first learn the wants, desires, and problems of your users. Then build a product to solve them.
Often data warehouses become the Juiceros of the data world in that they’re expensive, exquisitely designed machines that were built first and afterwards sought users.
Whether you're building a data warehouse or any other product, this is an important article to help you embrace product thinking.
Read more about product thinking

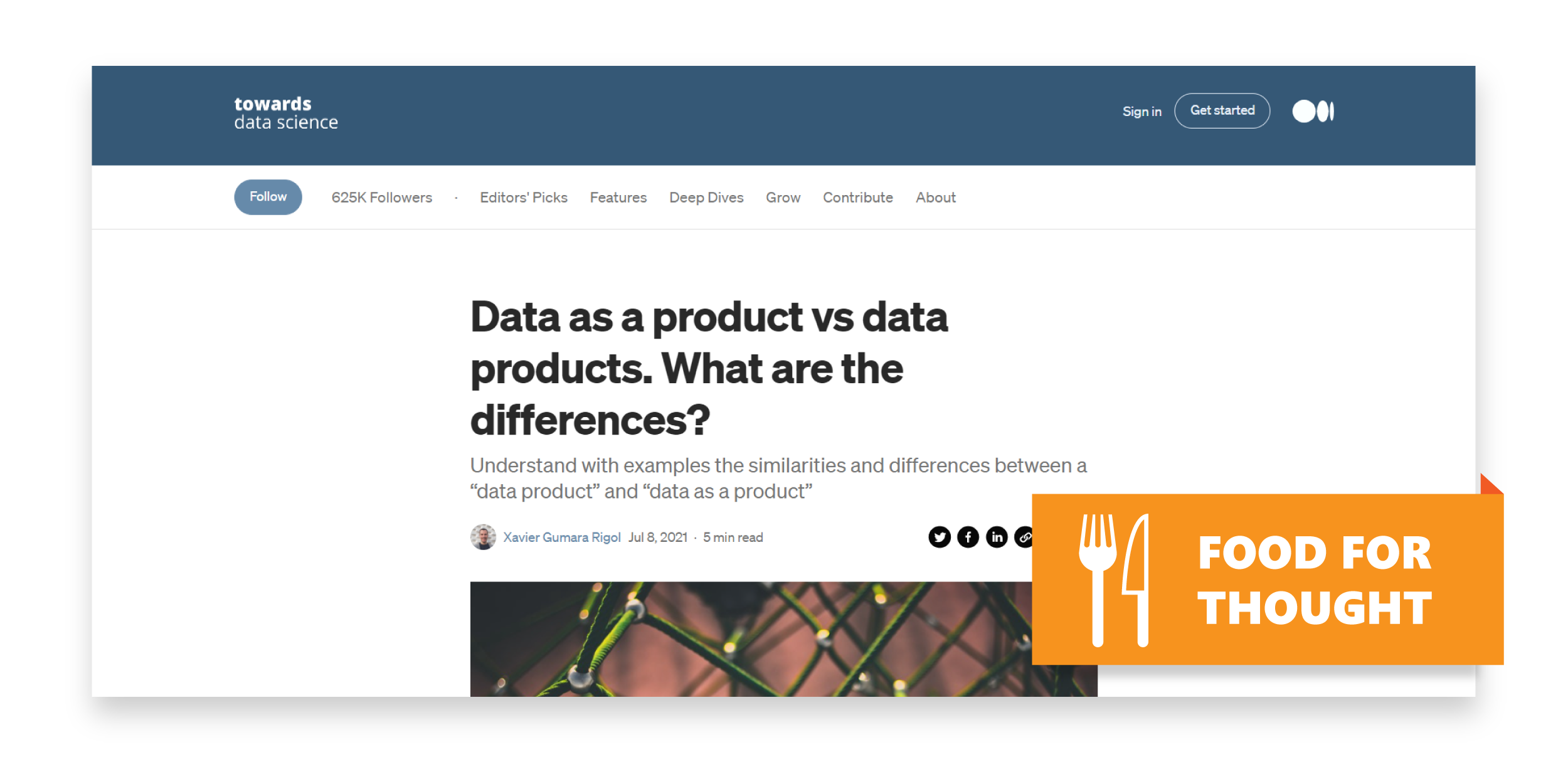
Data as a Product
In sales, there’s a concept known as friction. Friction is anything in the sales pipeline that slows down or complicates the process.
Imagine we have a candy vending machine located in an unlabeled room in the basement of an office building. To get into the room, you first must track down the custodian and ask for the key. You get to the machine and see that none of the choices on the vending machine are labeled. You have to look those up in a manual. If you want soda with your candy, well that vending machine is in a different room. It's logical that we can expect the soda machine to have few sales, if any.
Let’s apply the concept of friction to data. If a business-user wants to use data, they must first:
- Know the data exists
- Know what system holds the data
- Get access to the data
- Understand how to work with the system that holds the data
- Figure out which tables/columns have the data they need
- Know how to interpret codes and lingo in the data
- Know which transformations (filters, calculations, joins, etc.) are needed to make the data usable
The primary responsibility for a data platform should be to reduce these frictions as much as possible. Data as a product addresses this issue, so read these articles if you want to learn how to reduce friction.
Find out more about data as a product
What’s data as a product vs data products?


AWS Data Exchange for Amazon Redshift
In the old days, if a company was selling data, they’d usually send you a CSV file via email or FTP. More recently, companies have used REST APIs to provide data to customers. The latest development is a data exchange.
The phrase data exchange has been around for a while, but we’re using it in its modern context in data warehouses. All the major cloud data warehouse platforms are either built on or have access to object storage: AWS S3, Azure Blobs, GCP Cloud Storage. These are serverless simple to access data stores. To get access, you add permissions.
For example, in Snowflake you simply subscribe to a provider, and it instantly pops up in your list of datasets. No data movement needed. Snowflake brags about 800 datasets on their exchange, but, in the grand scheme, that isn’t a whole lot. It’ll be interesting to see if this concept takes off in the next few years.
Learn more about AWS Data Exchange

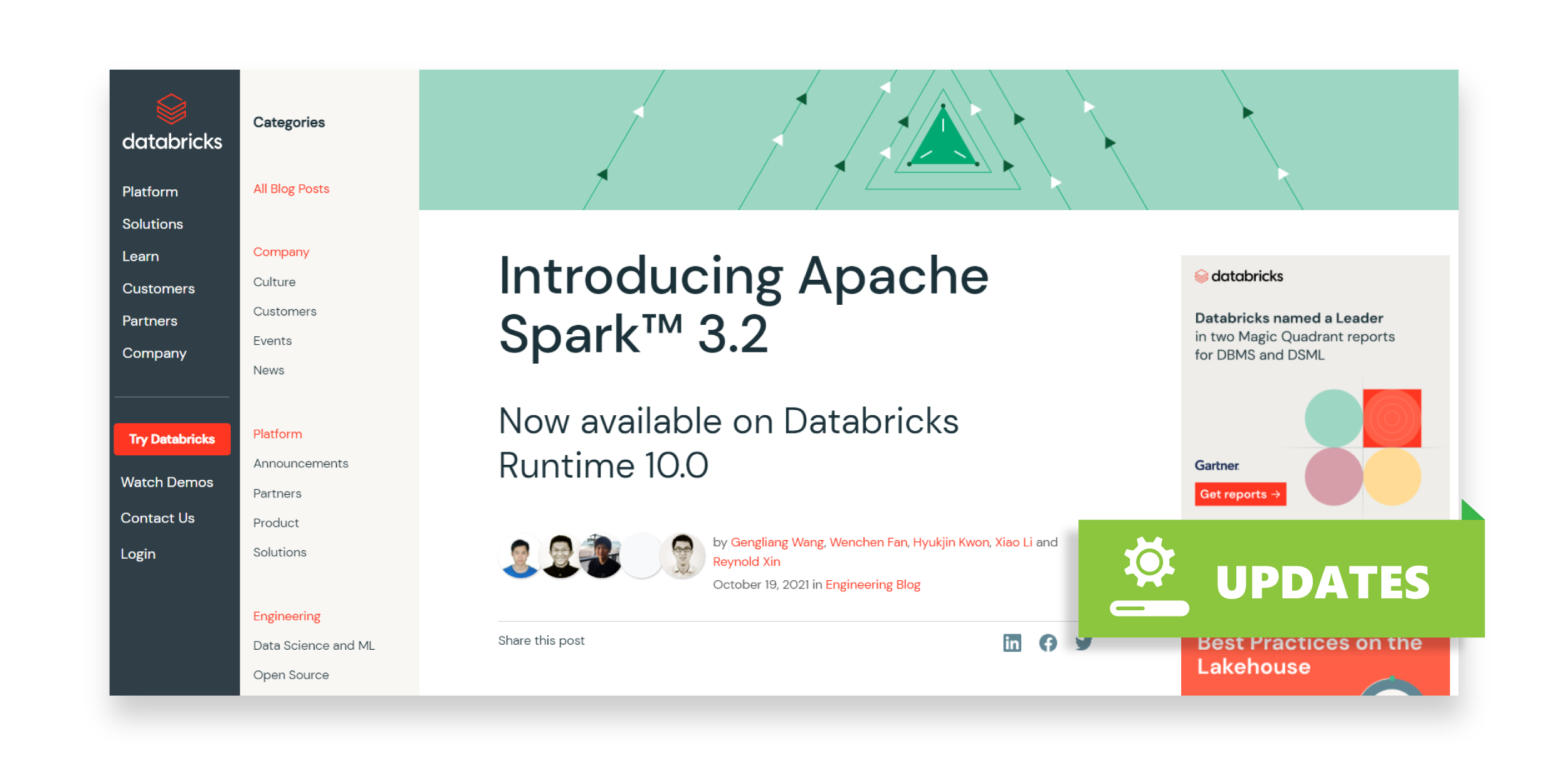
Apache Spark™ 3.2: Available on Databricks
Databricks (Apache Spark’s primary maintainer) is trying to position itself to compete with Snowflake and other cloud data warehouses. It introduced Apache Spark 3.2 in late 2021.
Spark is built on the JVM language Scala. For most of its life, Scala was the dominant and preferred language for writing Spark applications. In the last couple of years, thanks mostly to data scientists Python has become the dominant language. So Spark made a number of changes to make it more accommodating to Python users.
The most recent is Project Zen. One goal is to be able to migrate Pandas code to Spark with little friction. Apache Spark 3.2 brings a number of improvements to Spark SQL, and you’ll find the details below.


AWS Hero Writes: "9 Things I Freakin’ Love About Google Cloud Identity"
Many who work frequently with Google’s Cloud Platform will report that their security and organization are far superior to what Azure and AWS offer. This article explains why, and the appeal is that it's written by a long-time AWS Hero (expert) who began working with GCP.
Some of the main points: all security with humans is done with Google accounts. All machines use service accounts. It’s pretty easy. GCP’s resource hierarchy is far better than AWS or Azure. You can easily subdivide your company into folders and projects. Billing doesn’t have to line up with your project structure. Take time to read this one.
Read 9 things to love about GCP security
