When considering methods for storing information, data lakes and data warehouses are two solutions that will most likely come up for consideration. This can cause confusion for those unfamiliar with these concepts because they are often mistakenly used interchangeably, making it harder to recognize their differences.
Data lakes and warehouses are unique solutions that each serve distinct purposes and play their own crucial role in storing and managing data. In this article, we’ll set the record straight on the real meaning of data lakes and data warehouses, defining each term and exploring their key differences and similarities to help you understand the purpose of each and decide which is best for your organization.
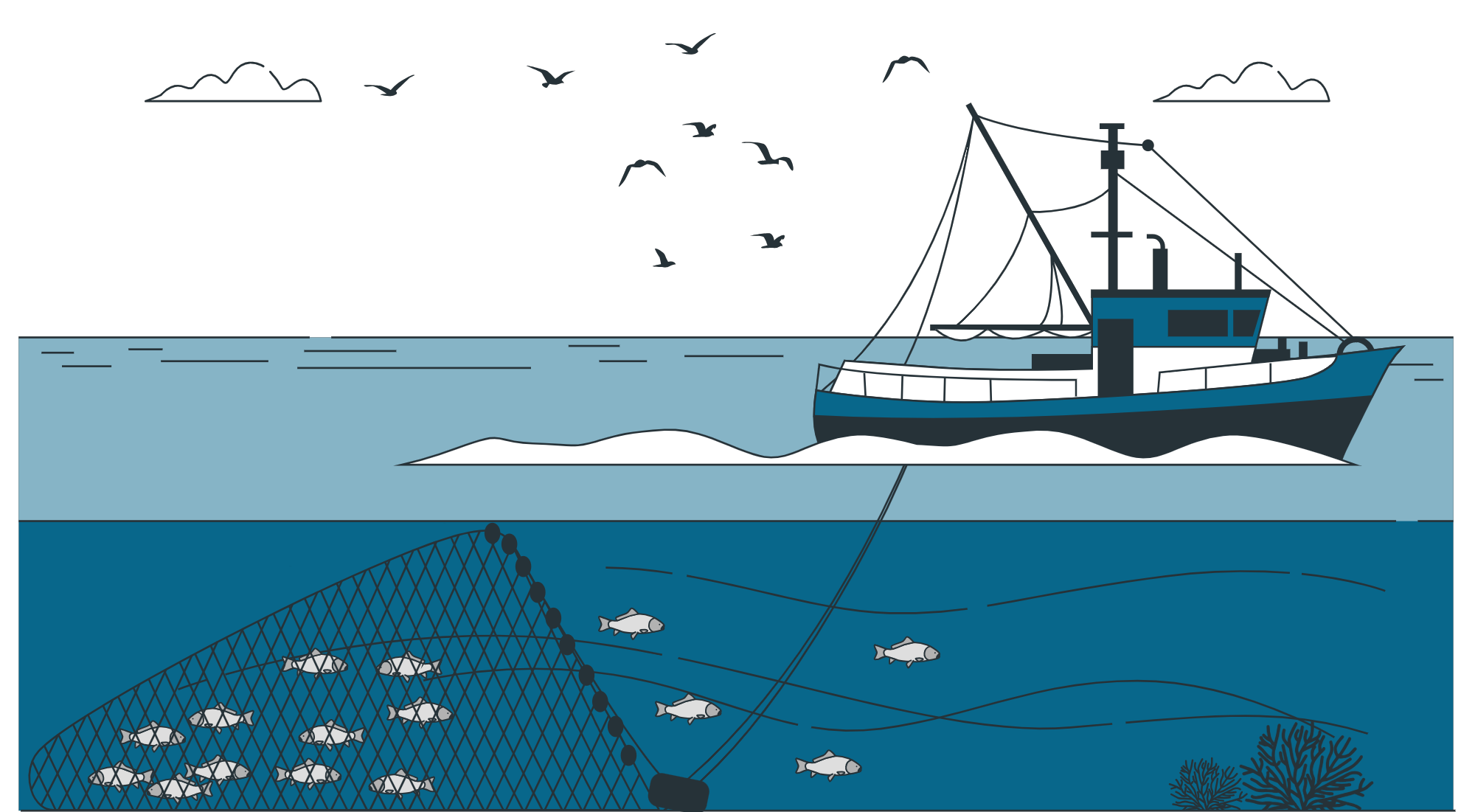
Data Lake
A data lake is a dynamic system or repository that stores large amounts of raw, unstructured, and structured data. Unlike traditional data storage systems, data lakes can accommodate data in its native form, offering users the flexibility to handle data in various formats. A data lake serves as a central hub for organizations to collect and store data without the need for extensive preprocessing, this can make the data more accessible and easier to update, but the complexity and lack of structure restricts who can understand and use this data. You can think of a data lake like a real lake, where multiple rivers and streams flow together to make one body of water, a data lake stores information that flows in from multiple sources and floats around.
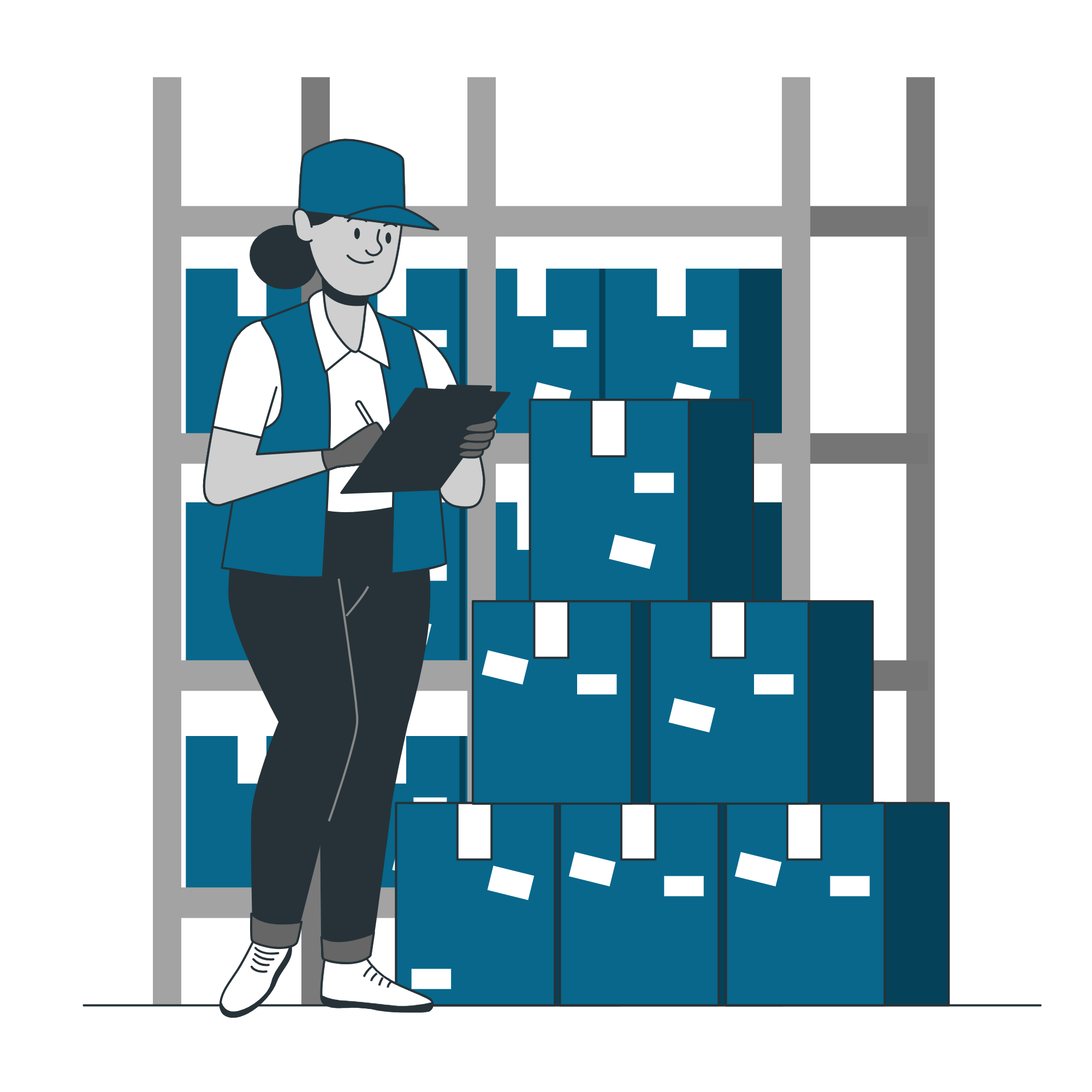
Data Warehouse
A data warehouse, on the other hand, is a structured storage system where data is organized and processed to support business intelligence and analytics. With a data warehouse, data is extracted from multiple sources, cleaned, restructured to a specific format, then organized and stored based on its predetermined purpose. In the same way, a data warehouse is like your local wholesaler – products or data flow in from multiple sources and are analyzed and sorted on shelves or in data marts based on their purpose or type.
Differences and Similarities
Let’s say you went to a local trade show. You set out a fishbowl instructing guests to drop their business cards in for a chance to win a raffle. All the cards are randomly mixed throughout the bowl with no rhyme or reason, there may even be a few of the same ones – this is a data lake. Now let’s say you take this fishbowl home and organize the cards, you remove duplicates and sort the info into a structured Excel Sheet where all the info is uniform – this is your data warehouse. This gives us a metaphorical idea of how data lakes and warehouses are different, but let’s look at the characteristics that make them unique.
Data Types and Structure
Data Lake: Data lakes can store both raw and processed data in its original format. Because data lakes support primarily unstructured data, they typically require a larger storage capacity, but raw data is more malleable and can be quickly analyzed. Because of the lack of structure and organization, it’s important to consider proper data quality strategies to prevent your data lake from turning into a data swamp.
Data Warehouse: Data warehouses only store purposefully structured, processed, and cleaned data. Because the information stored in a data warehouse is transformed and organized to serve a certain purpose, the data is easier to decipher but more difficult or costly to manipulate.
Processing
Data Lake: Data processing in a data lake typically uses ELT (Extract, Load, Transform) tools. Because data is loaded into the lake with the sole purpose of being stored, information is only processed for use when needed.
Data Warehouse: ETL (Extract, Transform, Transform) processes, are typically used for data warehouses to clean, filter, and structure data before being stored. Preprocessing can identify duplicates, errors, and unverified data before being loaded into the data warehouse, making accurate and reliable data accessible more quickly.
Use Cases
Data Lake: Data lakes are best for storing large volumes of data whose purpose has not yet been determined. The ability to easily manipulate and access large amounts of data makes data lakes most suitable for machine learning, data science, and data staging. It is important to remember that because in-depth analysis is needed to understand this data, data lakes are only practical if you have access to a data professional who can extract and interpret this data for business use.
Data Warehouse: Because the information stored in data warehouses has been processed to develop insights and inform decisions, they are optimal for business intelligence, reporting, and data analysis. Processed data can be used in charts, spreadsheets, tables and more to provide structured insights that most business users can comprehend, making it applicable for use across organizations where resources may be limited.
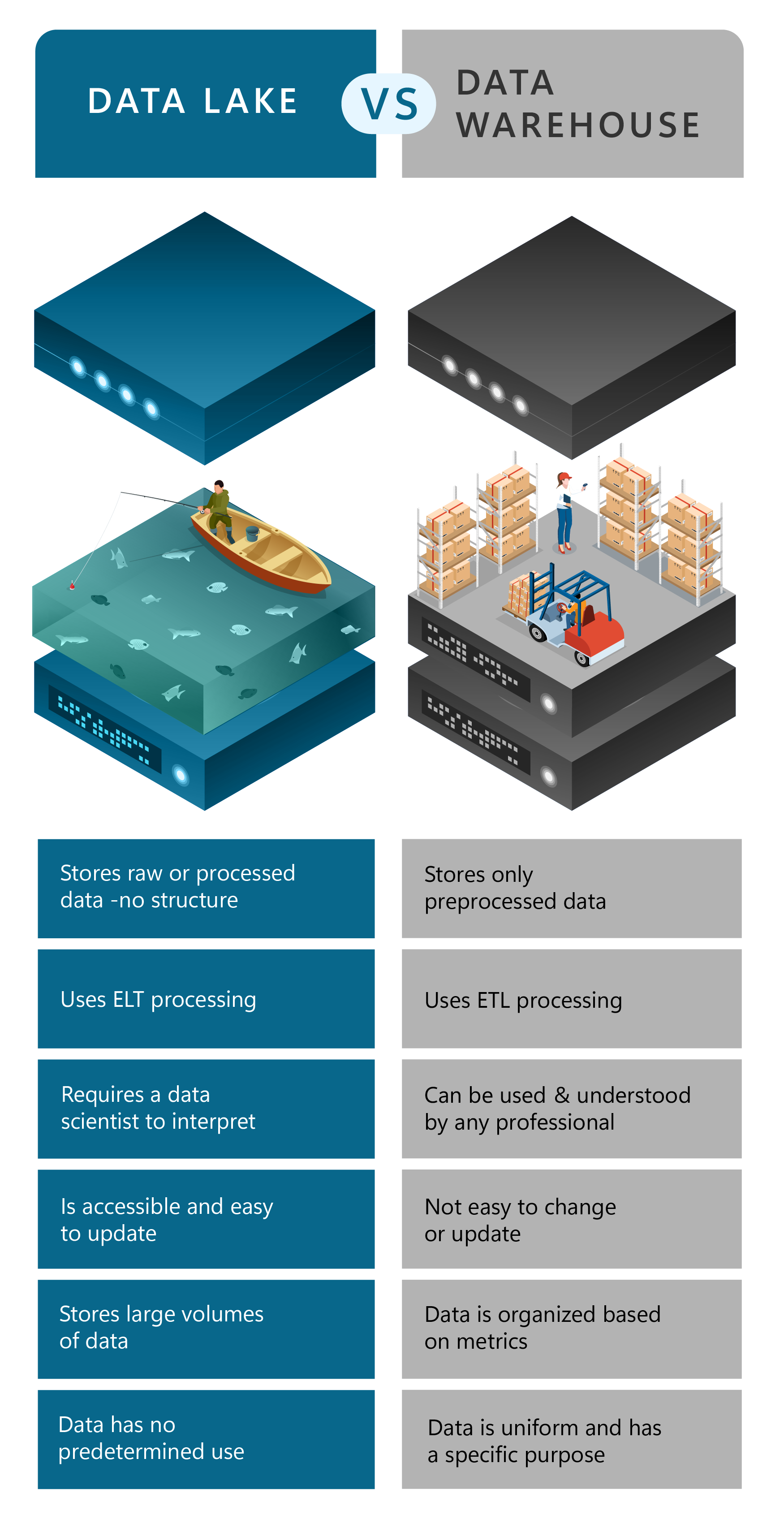
Data Lake vs Data Warehouse: What’s the Right Choice?
Now that we understand how truly different the purposes of data lakes and warehouses are, how can we determine which is the best fit for your organization? While data lakes provide the flexibility and capacity of raw and unstructured data, they’re not exactly easy to use or understand for everyone. On the other hand, data warehouses provide a meticulously organized repository of simplified data, making them easier to comprehend for more use, but typically at a higher cost.
The truth is most organizations need both to cover their full spectrum of data storage. A data lake will allow you to store large amounts of data at a low cost and provides flexibility to use this data for multitude of business cases. Data can then be loaded into one or several data warehouses for certain use cases or users.
While a data lake prioritizes storage volume over performance, a data warehouse prioritizes structure and accuracy, allowing business users to generate reports more efficiently. Harnessing the power of both will give your organization the ability to handle any amount of data, while still ensuring it is accessible, reliable, and comprehensible.
Onebridge has successfully executed data lake and warehouse migrations, automation, and accelerations for many organizations and we have the experience to help yours implement a data storage strategy that you can rely on. No matter where you are in your data journey, we can meet you are to manage and execute a data storage strategy that will meet your business needs. Connect with us today to learn how.